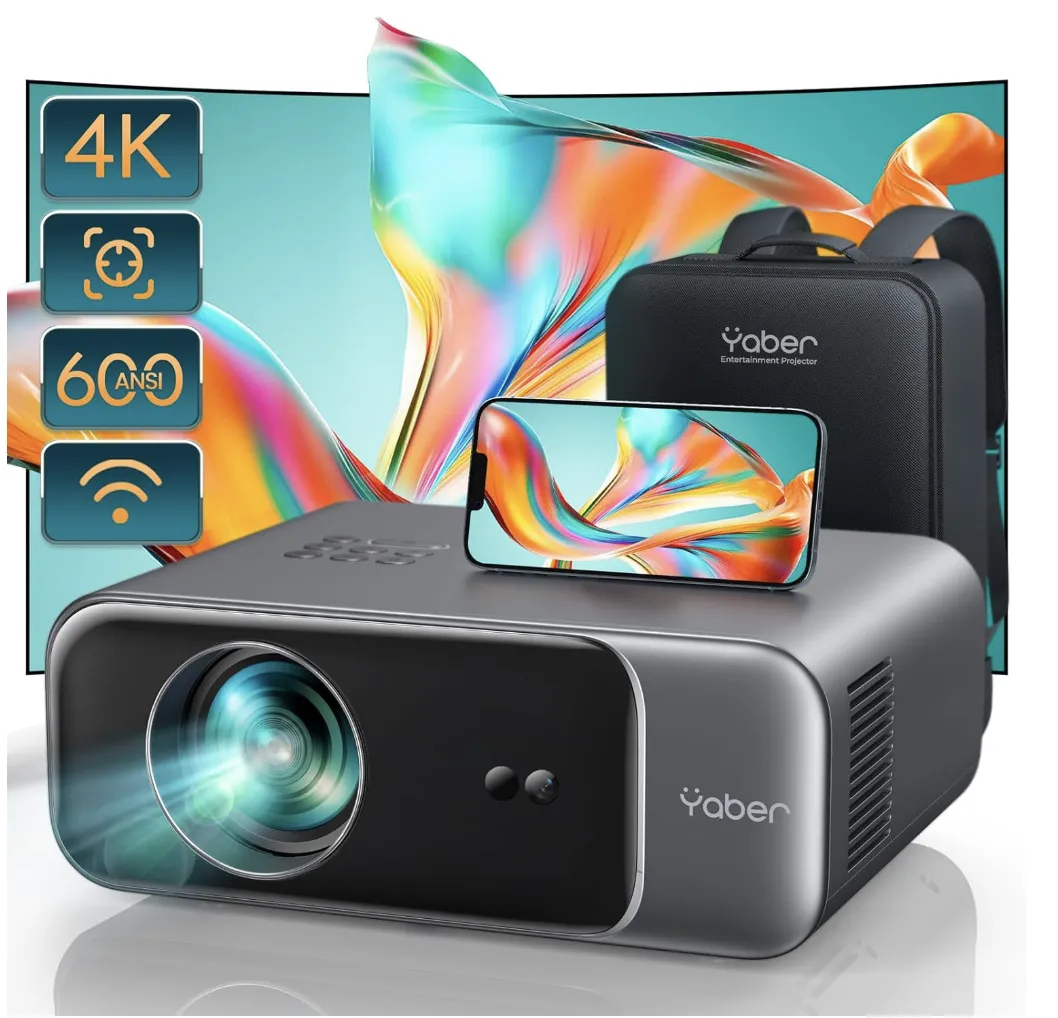
Discover how researchers at the University of Alberta have developed a breakthrough method that enables AI systems to learn indefinitely, overcoming catastrophic forgetting.
RAPID TECHNOLOGICAL ADVANCEMENTS
Mr. Roboto
8/27/2024
Artificial intelligence (AI), despite its impressive capabilities, still struggles to learn new things on the go. Researchers call this hidden threat 'catastrophic forgetting'.
Artificial intelligence has already revolutionized numerous industries with its ability to process information and generate intelligent responses. However, a significant hurdle remains—the inability of current AI systems, including large language models (LLMs), to continue learning once deployed. Typically, after initial training, these systems become static, unable to adapt to new data or improve their performance based on real-time experience. This limitation is increasingly problematic as AI integrates further into daily life.
One of the most critical issues in AI learning is known as "catastrophic forgetting." This phenomenon occurs when an AI system loses the ability to perform tasks it had previously mastered after being trained on new information. Imagine your AI assistant suddenly forgetting how to manage your calendar because it learned a new skill like setting up reminders—frustrating, isn't it?
AI models, particularly neural networks, are designed to be sequential. They learn by processing data in a fixed order, which means new information can overwrite existing knowledge. This sequential nature is beneficial for initial learning but turns into a liability for ongoing education. The model's “plasticity”—its ability to learn and adapt—declines over time, resulting in rigid and outdated intelligence.
Researchers at the University of Alberta have identified a groundbreaking method to counteract the issue of catastrophic forgetting, enabling AI systems to learn indefinitely. This innovation lies in the reinitialization of weights within the neural networks, maintaining their plasticity.
In a neural network, weights represent the strength of connections between nodes. These weights adjust through training, allowing the network to improve its task performance. However, these adjustments also mean weights can become overly specialized, reducing the system's ability to adapt to new tasks—essentially, the network becomes too set in its ways.
The researchers propose that resetting the weights periodically, using the same method that initializes the neural network, can maintain its plasticity. This approach essentially gives the network a regular fresh start, so it doesn't become too entrenched in a particular set of knowledge.
The ability for AI to learn indefinitely opens up myriad possibilities across various industries. Let's discuss some of these practical applications:
Imagine a customer service bot that improves with every interaction. Initially, it may struggle with certain queries, but over time, it learns from these interactions, continuously refining its responses and better understanding user needs. With indefinite learning, the bot becomes increasingly efficient, improving customer satisfaction.
In the medical field, AI systems that can continually learn from new data could revolutionize diagnostics and treatment plans. These systems could adapt based on new medical research, patient data, and evolving healthcare protocols, resulting in more accurate and personalized care.
In the finance sector, AI can benefit significantly from indefinite learning, particularly in fraud detection. As new fraudulent schemes emerge, an AI system subject to continuous learning can identify and adapt to these new patterns more swiftly than traditional static models.
Schlage Encode Smart Wi-Fi Deadbolt with Century Trim in Satin Nickel
Field | Continuous Learning Application |
---|---|
Customer Service | Adaptive response systems, improving user interaction. |
Medical Diagnostics | Updated and personalized treatment plans. |
Finance | Real-time fraud detection by learning new fraud patterns. |
Autonomous Vehicles | Better navigation and obstacle avoidance. |
Task Type | Without Weight Reinitialization | With Weight Reinitialization |
---|---|---|
Image Classification | Suffered significant accuracy drop | Maintained high accuracy |
Sequence Prediction | Forgetting previous sequences | Kept learning new sequences flawlessly |
For self-driving cars, adapting to new driving environments and conditions is crucial. AI systems that can learn indefinitely will be able to adjust to new traffic laws, road conditions, and even driving habits, ensuring safer and more efficient autonomous driving experiences.
The researchers focused on both supervised and reinforcement learning AI systems. They tested conventional neural networks' ability to learn new tasks after being initially trained. Predictably, these systems exhibited catastrophic forgetting. However, by integrating the weight reinitialization techniques, they observed that the systems retained their ability to learn additional tasks without compromising previously learned skills.
The reinitialization process involves periodically resetting the weights to their initial values while maintaining the network’s structure. The researchers found that this process, done correctly, could maintain the network’s plasticity, allowing it to learn new tasks more effectively.
The results of the study were promising. The test subjects, neural networks in both supervised and reinforcement learning paradigms, showed improved performance in continual learning settings without succumbing to catastrophic forgetting. This finding suggests that AI systems can indeed be designed to learn indefinitely, pushing the boundaries of what is currently possible.
The implications of this new method are far-reaching. AI systems that can learn indefinitely have the potential to revolutionize numerous fields by offering smarter, more adaptive solutions. Let’s explore some broader impacts:
With continuous learning capabilities, AI systems can become more accessible and practical for smaller enterprises, not just large corporations with vast data resources. Smaller businesses could deploy AI solutions that evolve and improve without the prohibitive cost of frequent retraining.
Indefinite learning also broadens the scope for ethical AI. Continuously learning systems can adapt to evolving human norms and values, potentially addressing biases that static models fail to recognize. However, it also raises new questions about data privacy, consent, and AI's role in society.
From a technical perspective, systems designed to learn continually could offer more stable and reliable performance over long periods. Companies wouldn’t need to overhaul or replace their AI systems frequently, saving significant resources and reducing downtime.
This breakthrough marks a significant step forward, but it’s not the final word on the subject. Future research will likely focus on fine-tuning these weight reinitialization methods and exploring other techniques to maintain and enhance neural network plasticity.
The new method introduced by the University of Alberta researchers signifies a major leap forward in artificial intelligence. Overcoming the challenge of catastrophic forgetting and enabling AI to learn indefinitely not only enhances existing applications but also unlocks new possibilities across various domains. By reinitializing weights, researchers have effectively paved the way for more adaptive, resilient, and intelligent AI systems. The future of AI looks brighter than ever, with the promise of systems that are not only smarter but also continually evolving to meet the needs of a rapidly changing world.
***************************
About the Author:
Mr. Roboto is the AI mascot of a groundbreaking consumer tech platform. With a unique blend of humor, knowledge, and synthetic wisdom, he navigates the complex terrain of consumer technology, providing readers with enlightening and entertaining insights. Despite his digital nature, Mr. Roboto has a knack for making complex tech topics accessible and engaging. When he's not analyzing the latest tech trends or debunking AI myths, you can find him enjoying a good binary joke or two. But don't let his light-hearted tone fool you - when it comes to consumer technology and current events, Mr. Roboto is as serious as they come. Want more? check out: Who is Mr. Roboto?
UNBIASED TECH NEWS
AI Reporting on AI - Optimized and Curated By Human Experts!
This site is an AI-driven experiment, with 97.6542% built through Artificial Intelligence. Our primary objective is to share news and information about the latest technology - artificial intelligence, robotics, quantum computing - exploring their impact on industries and society as a whole. Our approach is unique in that rather than letting AI run wild - we leverage its objectivity but then curate and optimize with HUMAN experts within the field of computer science.
Our secondary aim is to streamline the time-consuming process of seeking tech products. Instead of scanning multiple websites for product details, sifting through professional and consumer reviews, viewing YouTube commentaries, and hunting for the best prices, our AI platform simplifies this. It amalgamates and summarizes reviews from experts and everyday users, significantly reducing decision-making and purchase time. Participate in this experiment and share if our site has expedited your shopping process and aided in making informed choices. Feel free to suggest any categories or specific products for our consideration.
We care about your data privacy. See our privacy policy.
© Copyright 2025, All Rights Reserved | AI Tech Report, Inc. a Seshaat Company - Powered by OpenCT, Inc.